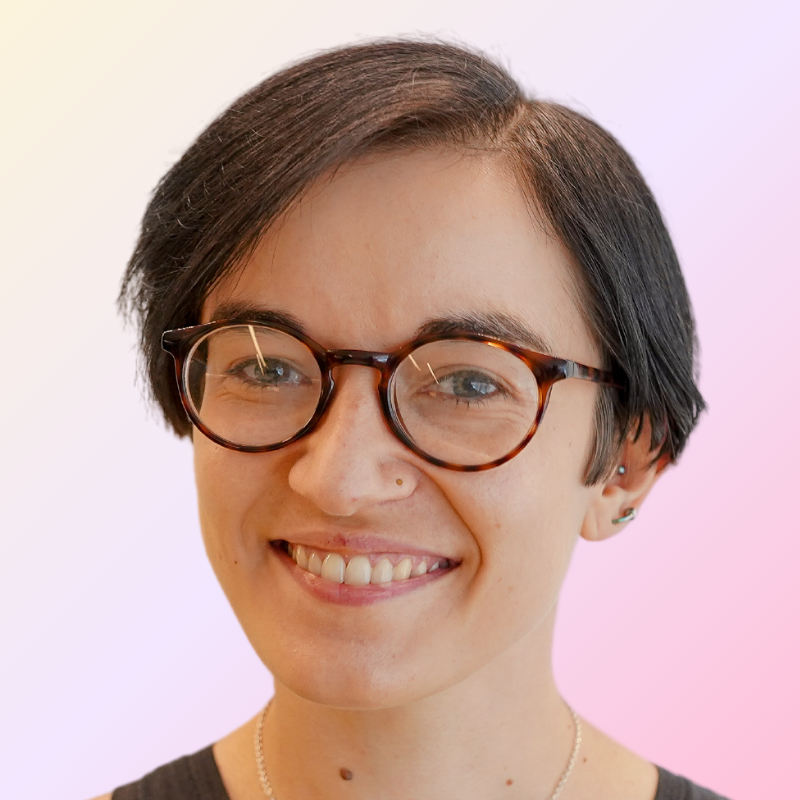
Designing AI Products: Adapting traditional Product Design skills to an AI company
.png)
There are many articles online on the subject of designing with AI, but the discipline of designing for AI products is very much still emerging.
Reflecting on my first six months as Lead Product Designer at 抖阴短视频 (a Google Ventures-backed AI legal assistant), here鈥檚 what I鈥檝e learned about:
- Applying a traditional Product Design skillset to designing AI-native products
- The new challenges that Product Designers face when designing for AI products
鈥
1. It鈥檚 complicated
Traditional product design focuses on (comparatively!) predictable, linear user journeys with clear outputs. Your product is now thinking, planning and acting independent of how you designed it. The specific actions of both the user and the AI remain uncertain. You have to anticipate and support their behaviours as best you can, without being able to fully predict them.
You鈥檒l gain a new ally in this labyrinthine new world: the Machine Learning Scientist or Engineer. Your work is massively interdependent. Often the Product Design and ML R&D phases are one and the same. The work you do together will be the highlight of your job, but you鈥檒l need to deepen your technical knowledge. You need understand of how AI systems work in order to be able to design for them.
鈥
2. Prompts matter
.png)
Better information from the user means better AI outputs. You need to figure out what kind of data the AI needs to do its job and then support the user to deliver it. You鈥檒l need to test both test what the user prompts are likely to be, and also what effect that information will have on the system itself and the outputs it gives.
User inputs are also an information goldmine! When thinking about how to improve Genie鈥檚 AI editor, we analysed anonymised data from users鈥 current prompts. Even when the system couldn鈥檛 yet perform some of the tasks, analysing unsuccessful user prompts revealed potential future features for our document editor.
.png)
鈥
3. Prototyping AI tools is different
To do more simple usability testing, you can still often get away with traditional Figma prototypes populated with example scenario text. However testing the AI system itself requires collaboration with ML teams to build functional AI prototypes.
When you go into user testing, even the ML scientists aren鈥檛 sure how the prototyped AI will behave. These can be tested using all your usual user research skills, but the uncertain nature of both the inputs and outputs means you should be prepared for the unexpected during testing!
.png)
鈥
4. Design for Trust
.png)
Trust (both in terms of security, and in the quality of AI outputs) is one of the core barriers to AI adoption. It鈥檚 particularly huge for us at 抖阴短视频. Getting lawyers to trust AI with their precious legal documents is no mean feat.
Strategies to positively impact users鈥 conscious sense of trust in AI range from . It鈥檚 a complex concept that touches every part of the user journey.
Thankfully, your product design skillset comes in handy here. Understand the points at which trust (or a lack thereof) has an impact when using your product. We鈥檝e user tested everything from our the security messaging on our Marketing website to the legal quality of our AI-suggested contract clauses. For example, the below messaging increased the number of first-time users uploading a legal contract for AI review by 17%!

鈥
4.a Trust is subconscious
It鈥檚 not just about trust and security in the traditional sense. The conscious and sub-conscious factors that influence a users鈥 feeling of trust include things like brand design, tone of voice, messaging consistency, the list goes on and on..
Familiar interfaces and interaction patterns, common to other existing products, increases users鈥 subconscious sense of trust. This lets you leverage the trust they have in the products they鈥檙e already using to make using an AI tool less scary.
Buttons, form fields and other UI elements should also work uniformly across the whole product as 鈥溾 decrease users鈥 expectation of risk.
鈥
5. Adapting your core design skills
Designers transitioning into AI products need to adapt to a more uncertain, complex landscape. To make the leap, you鈥檒l need to understand the key challenges specific to AI and increase your technical understanding.
This being said, the core design thinking skillset is still absolutely relevant when designing for AI products. All of the above can be learnt on the job as long as you stay flexible and curious.
鈥
Just in case you were wondering, this was written by a human.
鈥
Interested in joining our team? Explore career opportunities with us and be a part of the future of Legal AI.
鈥